The Impact of Machine Learning on Financial Services
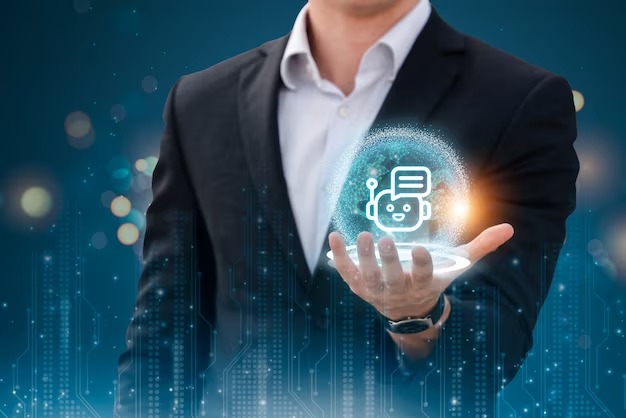
Introduction
The financial services industry stands at the forefront of technological innovation, with machine learning (ML) being one of the most significant disruptors. ML, a subset of artificial intelligence, involves the use of algorithms and statistical models to enable computers to improve their performance on a specific task with data, without being explicitly programmed. Its applications in financial services are vast and varied, offering unprecedented efficiencies, insights, and capabilities. This article explores the profound impact of machine learning on various aspects of financial services, from banking and investments to risk management and customer service.
Understanding Machine Learning
At its core, machine learning analyzes large volumes of data to identify patterns, make decisions, and predict outcomes. It’s particularly well-suited to the financial services sector, which relies heavily on data and precision for decision-making. ML can process complex, voluminous, and diverse data sets more efficiently and accurately than traditional methods.
Applications of Machine Learning in Financial Services
- Risk Management: ML algorithms can predict loan defaults, identify fraudulent transactions, and assess credit risk with greater accuracy. By analyzing historical data, customer behavior, and market trends, financial institutions can make more informed decisions and mitigate risks more effectively.
- Algorithmic Trading: In the investment sector, ML is used for algorithmic trading, where it can analyze market data and execute trades at optimal times. ML algorithms can also identify new trading opportunities by recognizing complex patterns and trends in market data.
- Personalized Banking: ML enables personalized banking experiences by analyzing customer data to offer customized products, advice, and services. It can identify upsell and cross-sell opportunities, optimize pricing strategies, and provide personalized financial advice.
- Fraud Detection and Prevention: ML improves the ability to detect and prevent fraudulent activities by recognizing anomalies and patterns indicative of fraudulent behavior. It continuously learns and adapts to new fraudulent tactics, making financial systems more secure.
- Customer Service: ML powers chatbots and virtual assistants that provide customers with instant, 24/7 support. These AI-driven tools can handle a range of queries, improve customer engagement, and free up human staff to focus on more complex issues.
- Regulatory Compliance: Financial institutions use ML to navigate the complex regulatory landscape more efficiently. ML can monitor transactions, detect anomalies, and ensure compliance with various regulatory requirements, reducing the risk of costly fines and reputational damage.
The Impact of Machine Learning
- Enhanced Efficiency and Productivity: ML automates routine tasks, processes large volumes of data quickly, and reduces the likelihood of human error, leading to significant improvements in efficiency and productivity.
- Improved Decision Making: With its ability to analyze vast amounts of data and learn from outcomes, ML supports better decision-making in financial services. It offers deeper insights and more accurate predictions, enabling financial professionals to make more informed and strategic decisions.
- Innovation and Competitive Advantage: Institutions that effectively harness ML can develop innovative products and services, deliver superior customer experiences, and gain a competitive edge in the market.
- Cost Reduction: By automating tasks and improving accuracy, ML can help financial institutions reduce operational costs and losses due to errors or fraud.
Challenges in Implementing Machine Learning
While the benefits of ML in financial services are clear, its implementation comes with challenges:
- Data Privacy and Security: Handling sensitive financial data requires robust security measures and compliance with data protection regulations. Ensuring the privacy and security of data used in ML models is paramount.
- Quality and Bias of Data: The accuracy of ML models depends on the quality and representativeness of the data they’re trained on. Biased or poor-quality data can lead to inaccurate predictions and discriminatory outcomes.
- Complexity and Explainability: ML models, especially deep learning models, can be highly complex and difficult to interpret. This “black box” nature can be problematic in financial contexts where explainability is crucial for trust and regulatory compliance.
- Integration and Change Management: Integrating ML into existing systems and processes can be challenging. It requires significant investment, change management, and upskilling of staff.
The Future of Machine Learning in Financial Services
Looking ahead, machine learning will continue to transform financial services in profound ways:
- Continuous Learning and Improvement: As ML algorithms are exposed to more data over time, they will become even more accurate and sophisticated, leading to continuous improvements in financial services.
- Greater Personalization: The future will see even more personalized and customer-centric financial products and services, driven by deep learning about individual preferences, behaviors, and needs.
- Ethical AI and Responsible Use: There will be a growing focus on developing ethical AI frameworks and ensuring the responsible use of ML, balancing innovation with fairness, privacy, and security.
- Collaboration and Ecosystems: Financial institutions will increasingly collaborate with fintech startups, technology firms, and each other to leverage ML and other emerging technologies, creating dynamic ecosystems of innovation.
Conclusion
Machine learning is reshaping the financial services industry by enabling more accurate risk management, personalized banking, efficient fraud detection, and much more. As institutions navigate the challenges and embrace the opportunities presented by ML, they will need to focus on ethical use, data quality, and continuous improvement. The integration of machine learning into financial services is not just a trend; it’s a fundamental shift that will continue to drive innovation, efficiency, and competitiveness in the sector for years to come. As we move forward into an increasingly data-driven world, the role of machine learning in financial services will only grow, shaping the future of finance in the digital age.
(Nominate Now : Join us to spotlight your achievements! Be part of the elite in the business and finance community. Exciting opportunities await!)