Ethical Challenges in AI Implementation across Industries
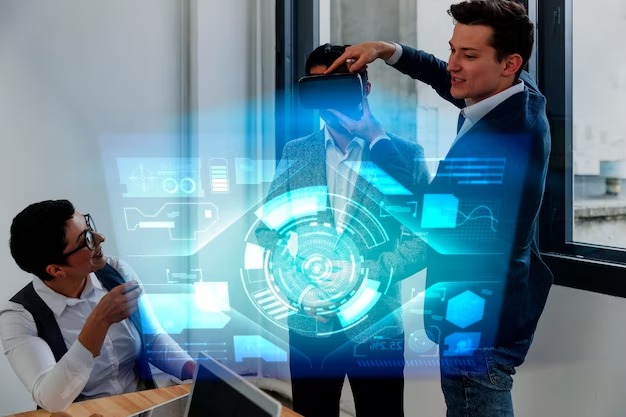
Introduction
Artificial Intelligence (AI) has rapidly evolved from a speculative science fiction concept into a core driver of modern industry. Its implementation spans a broad spectrum of sectors including healthcare, finance, retail, and more, providing efficiencies and capabilities that were previously unattainable. However, as AI systems become more integral to operations and decision-making, the ethical implications of their use have come into sharp focus. This article delves into the ethical challenges posed by AI implementation across industries and discusses how businesses, regulators, and the AI community can address these concerns.
Understanding the Ethical Implications of AI
The ethical challenges of AI are complex and multifaceted, often involving considerations of privacy, fairness, accountability, transparency, and the societal impact. As AI systems are designed to make decisions or assist in making decisions, the ethics of how these decisions are made, and on what basis, are critical.
- Bias and Discrimination: AI systems are only as good as the data they are trained on. Biased data can lead to biased decisions, potentially discriminating against certain individuals or groups in areas like job recruitment, loan approvals, or criminal sentencing.
- Privacy: AI’s ability to collect, analyze, and utilize vast amounts of data raises significant privacy concerns. There’s a risk of sensitive personal information being exposed, misused, or even stolen.
- Transparency and Explainability: Many AI systems, particularly those involving deep learning, are often described as “black boxes” because the way they make decisions is not transparent. This lack of explainability can be problematic, especially in critical applications like healthcare or criminal justice.
- Job Displacement: The automation capabilities of AI are leading to fears of widespread job displacement across various sectors. While AI can increase efficiency and reduce costs, the ethical implications of massive job losses are a significant concern.
- Accountability: When AI systems make wrong decisions, determining accountability can be challenging. Questions about who is responsible – the developers, the users, or the AI itself – are difficult to answer.
- Dependence and Autonomy: Over-reliance on AI systems can erode human skills and autonomy. In critical sectors, this can lead to situations where humans are unable to take over if the AI fails.
Ethical Challenges in Specific Industries
Each industry faces unique ethical challenges when it comes to AI implementation:
- Healthcare: In healthcare, AI promises to improve diagnostics, treatment planning, and patient monitoring. However, issues around patient data privacy, the need for consent, and the implications of misdiagnosis or biases in treatment recommendations are significant.
- Finance: In finance, AI is used for credit scoring, fraud detection, and automated trading among other applications. Ethical concerns include transparency in decision-making, data privacy, and the potential for systemic risks or market manipulation.
- Automotive: As the automotive industry moves towards autonomous vehicles, the ethical considerations multiply. Decisions around safety, the potential for accidents, and the programming of “ethical” responses in crash scenarios are profoundly complex.
- Retail and Marketing: In retail, AI is used for personalized marketing, inventory management, and customer service. Ethical issues involve the extensive collection of consumer data, privacy concerns, and the manipulation of consumer behavior.
Addressing the Ethical Challenges
Tackling the ethical challenges of AI requires a multi-faceted approach:
- Develop Ethical Guidelines and Standards: Industry leaders, ethicists, and regulators should collaborate to develop guidelines and standards for ethical AI. These should cover aspects like fairness, transparency, privacy, and accountability.
- Promote Transparency and Explainability: Efforts should be made to make AI systems as transparent and explainable as possible. This could involve developing new types of AI that are inherently more interpretable or creating standards for explainability.
- Ensure Diverse and Unbiased Data: To combat bias in AI, it’s crucial to use diverse and representative data sets. Regular auditing and testing for bias should be standard practice.
- Implement Robust Data Privacy Measures: Protecting personal data is crucial. This involves not only secure data practices but also considerations around what data is collected and how it is used.
- Foster Public Awareness and Education: Educating the public about the benefits and risks of AI, as well as their rights when it comes to AI decision-making, is essential. This also involves training the workforce to adapt to an AI-driven future.
- Regulatory Oversight: Governments and international bodies should play a role in regulating the use of AI, ensuring that it adheres to ethical standards and protecting citizens from potential harms.
Conclusion
The rapid advancement of AI across industries presents not only opportunities but also significant ethical challenges. Addressing these challenges is not straightforward and requires the concerted effort of all stakeholders – businesses, regulators, ethicists, and the public. As we continue to integrate AI into the fabric of industry, ensuring that it is developed and used ethically will be crucial to its success and acceptance. By proactively addressing the ethical considerations of AI, we can harness its full potential while safeguarding against its risks, leading to a future where AI contributes positively to society in a responsible and ethical manner.
(Nominate Now : Join us to spotlight your achievements! Be part of the elite in the business and finance community. Exciting opportunities await!)