Artificial Intelligence and Machine Learning in the Time of COVID-19
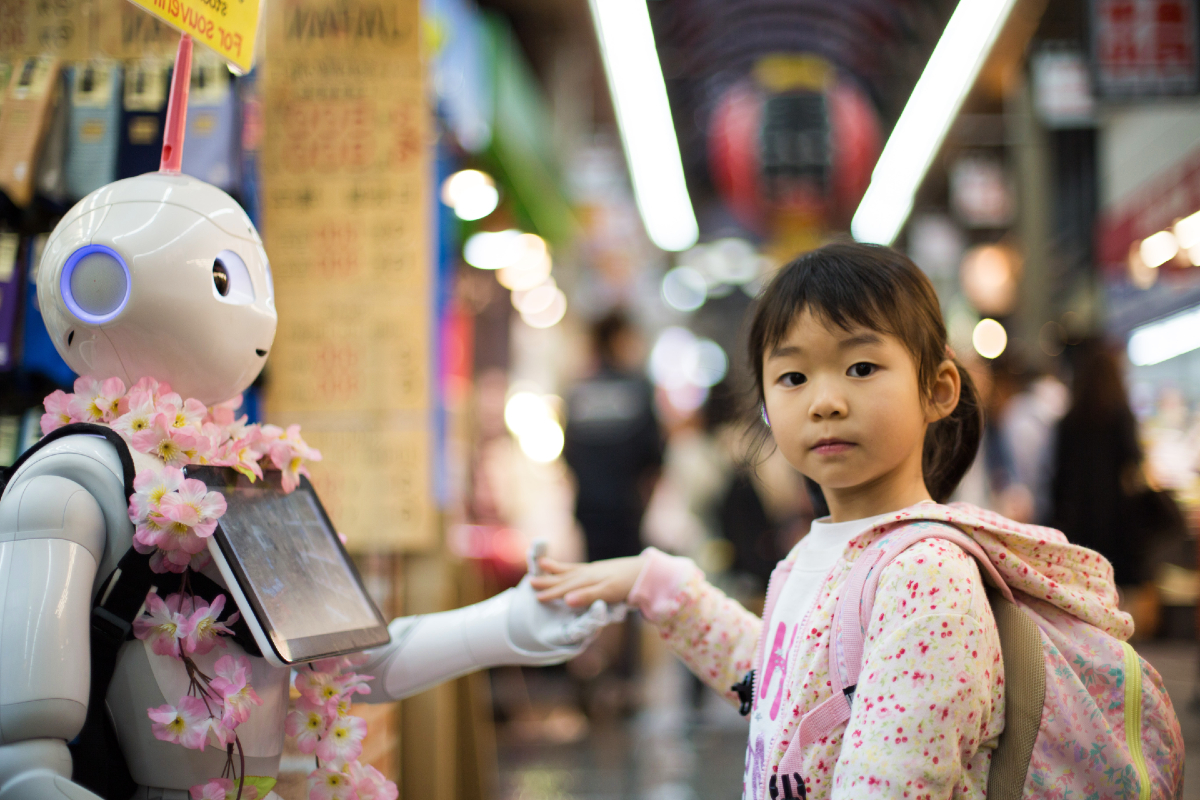
Researchers are finding new uses for artificial intelligence and machine learning during the pandemic, but they are not a silver bullet.
The COVID-19 pandemic has caused us major setbacks, but artificial intelligence and machine learning can help fill some of these gaps. By learning from artificial intelligence’s successes and consequences, we can develop responsible, data-driven solutions to help us through the current crisis.
What is the potential of artificial intelligence and machine learning? Artificial Intelligence refers to a number of ways machines are built to perform with human-like intelligence. Under its broad field is a subset called machine learning, which involves the processing of massive amounts of data combined with sophisticated algorithms into machines so they can “learn” specific tasks automatically. This helps us pinpoint patterns or make decisions from a sea of information.
In the past, artificial intelligence and machine learning have proven effective in various sectors (including healthcare) by improving old systems and fast-tracking innovation.
For example, the voice-powered app, Suki, uses artificial intelligence to help medical practitioners record clinical notes. Using a subset of machine learning called natural language processing, Suki is able to learn and adapt to the style and preferences of the practitioner and improve with every use. The system can also link to a hospital’s electronic health records and create prescriptions based on patterns and dosages–the doctor just needs to review and confirm the results. Suki’s website boasts a 100% accuracy rate, a reduction of documentation time by about 76%, and is currently used in about 85 sites in the United States.
In the current context, an artificial intelligence tool developed by New York University researchers is using predictive analytics. This tool finds patterns among patients with early COVID-19 symptoms and identifies who are more likely to get extremely sick. So far, the tool has had a 70-80% success rate using data from 53 patients. Currently in its experimental stage, the researchers are making further developments by expanding the data and improving accuracy.
As we learn from best practices and explore artificial intelligence in new contexts, we must also learn from past challenges.
Artificial intelligence and machine learning are not silver bullet solutions.
Artificial intelligence solutions are far from perfect. They can cost a lot of money, with complex algorithms and massive datasets needed. This might not be a problem for big companies with more skills and resources, but it could exclude small start-ups from making significant contributions.
The complexity of algorithms has also become a challenge. Infamously known as a “black box”, this refers to how we don’t always know how machines come to the conclusions they do. Others call this an “intellectual debt”, wherein we are able to find solutions to problems often without understanding the process. If left to grow, these unknowns could bring bigger problems in the future.
Another concern is the replication of bias. Facial recognition and natural language processing have been contested for “learning” racial and gender biases, from recognizing white skin more easily to attributing gender to certain jobs. Experts have also pointed out that the problem lies in how developers build systems as “objective truths”, when humans will always come from a limited perspective and may inadvertently feed this into machines which further build artificial intelligence and machine learning models that inherit these perspectives.
Artificial intelligence and machine learning are not silver bullet solutions. Our problems are increasingly complex and answers aren’t clear. Mitigating these risks ultimately circles back to our motivations for developing with artificial intelligence and machine learning.
In the development sector, each decision can impact the lives of the most vulnerable. Do we develop solutions with ethics and practicality, or is it for the sake of innovation? Are our processes transparent or do we keep others in the dark? Are we aware of the biases we feed into our machines, and can we continue to change them with the users in mind?
These questions should center the way we position artificial intelligence-driven solutions, especially during these challenging times. Now more than ever, we must be open to robust methods for data gathering and analysis to better adapt to our changing environment. If properly developed, artificial intelligence and machine learning can help us do that.
This is the first blog of a series by the Asian Development Bank and Thinking Machines Data Science, Inc., that will explore how we can responsibly use big data, artificial intelligence, and machine learning during this crisis to craft responsible solutions for development.